Improving outcomes for pregnant Bangladeshi women with gestational diabetes
Sumaia Sabouni, a recent PhD student based in Biomedical Science at the University of Bradford, is developing and piloting smartphone technology to enable women with gestational diabetes in Bangladesh to improve their pregnancy and diabetes outcomes with less clinical intervention.
Sumaia started her interdisciplinary PhD in June working alongside Professor Anne Graham from Chemistry and biosciences in the Faculty of Life Sciences, Professor Rami Qahwaji from Computing in the Faculty of Engineering and Informatics, and Professor Abigail Locke, from Psychology in the Faculty of Management, Law and Social Sciences.
Sumaia, a Software Engineering graduate with a year of experience working in the development and testing of enterprise level storage systems with IBM on placement in Manchester, applied for her PhD following a short teaching career in cyber security at the University of Bradford. She is passionate about teaching and research.
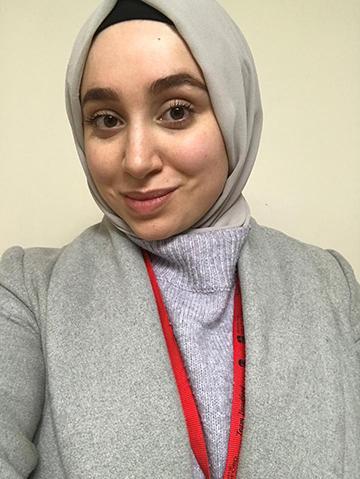
Sumaia Sabouni
I realised that I enjoyed academia far greater than I enjoyed working in industry. There is something truly rewarding about expanding the limits of human knowledge even a little on a particular topic and then transferring this knowledge to other people, whether that is through teaching or research.
Sumaia Sabouni
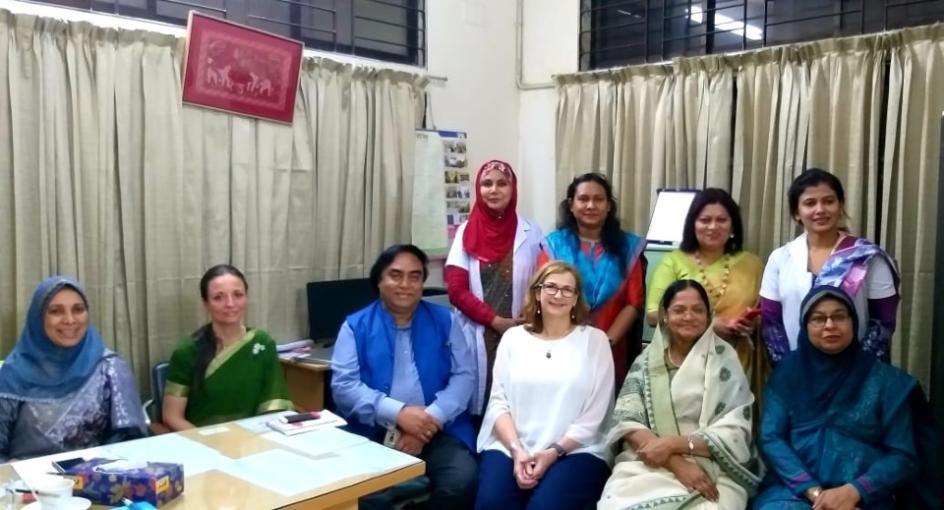
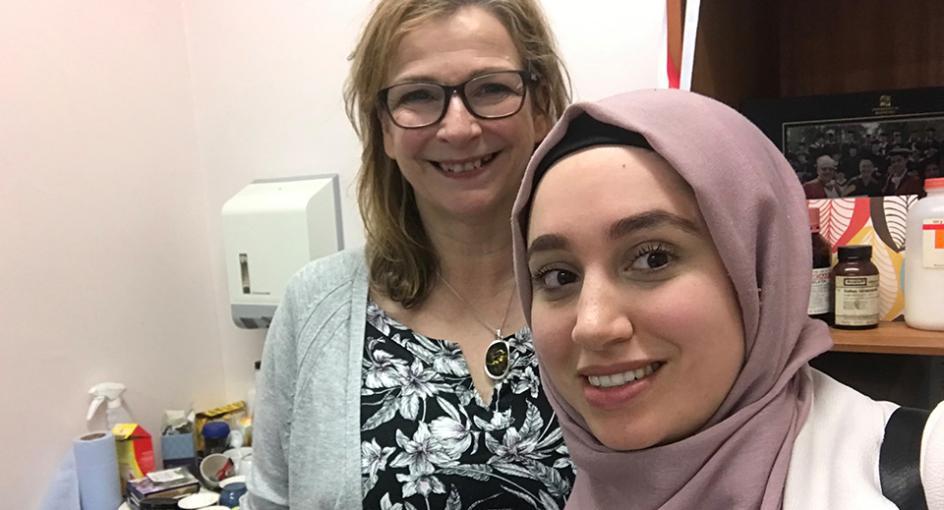
Sumaia’s study is called ‘Health Opportunity for Patient Empowerment in Gestational Diabetes (HOPE-GDM)’ and involves developing a clinical decision support system for Gestational Diabetes Mellitus (GDM) which can aid both clinicians and patients in managing the condition. Clinicians benefit by receiving preliminary evaluations from the system about the patient’s medical state, and patients benefit through healthcare plan adjustments relating to diet and exercise.
Gestational diabetes Mellitus (GDM) is one of the most common pregnancy complications accounting for 90% of all cases of diabetes in pregnancy. It can have numerous adverse effects on both mother and baby. There is also a lack of internationally accepted uniformity when it comes to screening, diagnosis and care for GDM.
One of the effective ways of managing diabetes in general is by empowering patients through education and awareness about their condition. This can be seen in several studies, such as a recent Bangladeshi pilot clinical study which involved hospital admission and included an educational package to give patients control in dietary and exercise habits and instructions on the benefits of blood glucose sampling and how effective interpretation could manage the disease. The study demonstrated a reduced number of mothers suffering pregnancy complications, increased the number of foetuses born within normal weight ranges, amongst other results. Despite these promising outcomes, the model requires an increased clinical workload and has economic costs. These combine to prevent its expansion to multiple centres across Bangladesh and India and are especially problematic in more rural areas.
Adoption of telemedicine is proposed to have benefits in diabetes care in pregnancy and provides a potential solution. Various telemedicine systems have been developed that harness GDM data to advance our knowledge and understanding of the condition. Such systems dealt with predicting and diagnosing gestational diabetes, calculating the risk of its transition to type 2 diabetes and managing the condition. However, many telemedicine systems in GDM are lacking in terms of usability and real-time user feedback. Additionally, such systems collect large amounts of data from their users, which must then be analysed by healthcare providers, potentially increasing their workload.
Therefore, in order for such systems to aid clinicians effectively, intelligent decision support capabilities must be incorporated. Decision support systems (DSS) use machine learning methods to analyse data, detect patterns, and uncover knowledge from data. DSSs are used to diagnose conditions, make recommendations to healthcare providers, adjust patient care plans, etc.
Sumaia’s project will collect information from the clinicians to establish how to obtain successful outcomes, interview patients to ensure the technology meets their needs, develop a machine learning augmented telemedicine intervention, and pilot the technology in a clinical trial. This will automate crucial labour intensive elements of the GDM patient service previously provided.